Basic concepts for omics data analysis
This page features the important fundamentals and concepts for omics data analysis.
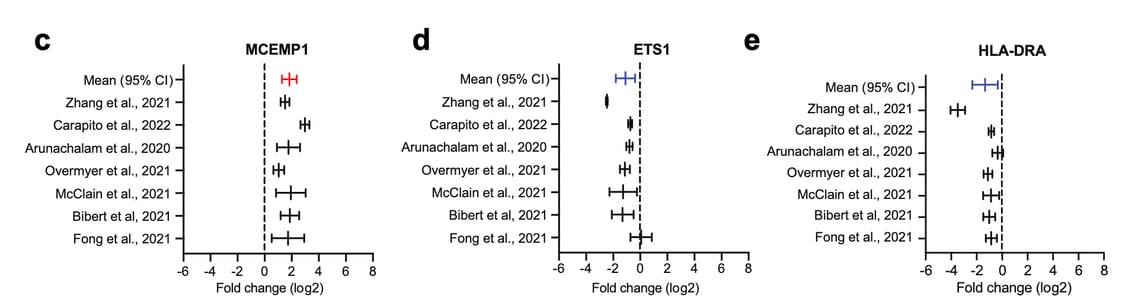
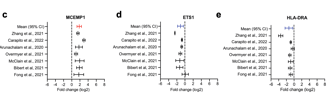
Analysing data between batches
Doing comparisons after an experiment is rather straightforward. But do you know how to compare data between different experiments? This is common with meta-analysis, where researchers are interested in comparing condition X vs condition Y across multiple cohorts.
An example is our research published recently in eBioMedicine, where we were interested to identify transcripts that were consistently dysregulated between severe and mild COVID-19 across different studies.
Some bioinformaticians may choose to do batch correction, but current batch correction methods are imperfect. If you have a common reference point, why not consider comparing fold-change values between studies instead?
For instance, in our research study stated above, we calculated fold-change values (severe vs mild COVID-19) across the different studies and identified two genes, MCEMP1 and HLA-DRA to be the most consistent biomarkers to discriminate severe from mild COVID-19 patients.
DO NOT just combine the raw values from multiple studies for comparisons, as there are many confounding factors that can influence the value of raw signals.
In conclusion, fold-change is a direct, simple and effective method to compare data between experiments. It also allows you to better estimate the effect size, which is the magnitude of differences between comparisons. As an example, check out Figure 1c-e from our publication (shown above).